Graphic:
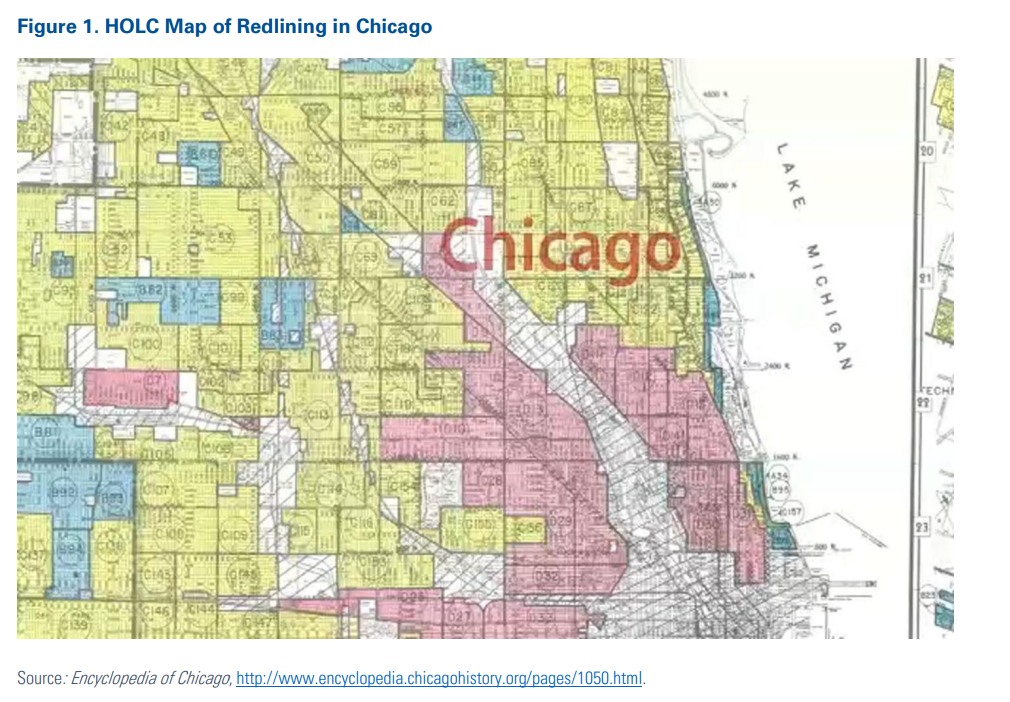
Excerpt:
This research paper’s main objective is to inspire and generate discussions
about algorithmic bias across all areas of insurance and to encourage
actuaries to be involved. Evaluating financial risk involves the creation of
functions that consider myriad characteristics of the insured. Companies utilize
diverse statistical methods and techniques, from relatively simple regression
to complex and opaque machine learning algorithms. It has been alleged that
the predictions produced by these mathematical algorithms have
discriminatory effects against certain groups of society, known as protected
classes.
The notion of discriminatory effects describes the disproportionately adverse
effect algorithms and models could have on protected groups in society. As a
result of the potential for discriminatory effects, the analytical processes
followed by financial institutions for decision making have come under greater
scrutiny by legislators, regulators, and consumer advocates. Interested parties
want to know how to quantify such effects and potentially how to repair such
systems if discriminatory effects have been detected.
This paper provides:
• A historical perspective of unfair discrimination in society and its impact
on property and casualty insurance.
• Specific examples of allegations of bias in insurance and how the various
stakeholders, including regulators, legislators, consumer groups and
insurance companies have reacted and responded to these allegations.
• Some specific definitions of unfair discrimination and that are interpreted
in the context of insurance predictive models.
• A high-level description of some of the more common statistical metrics
for bias detection that have been recently developed by the machine
learning community, as well as a brief account of some machine learning
algorithms that can help with mitigating bias in models.
This paper also presents a concrete example of an insurance pricing GLM
model developed on anonymized French private passenger automobile data,
which demonstrates how discriminatory effects can be measured and
mitigated.
Author(s): Roosevelt Mosley, FCAS, and Radost Wenman, FCAS
Publication Date: March 2022
Publication Site: CAS