Link:https://astralcodexten.substack.com/p/ivermectin-much-more-than-you-wanted
Graphic:
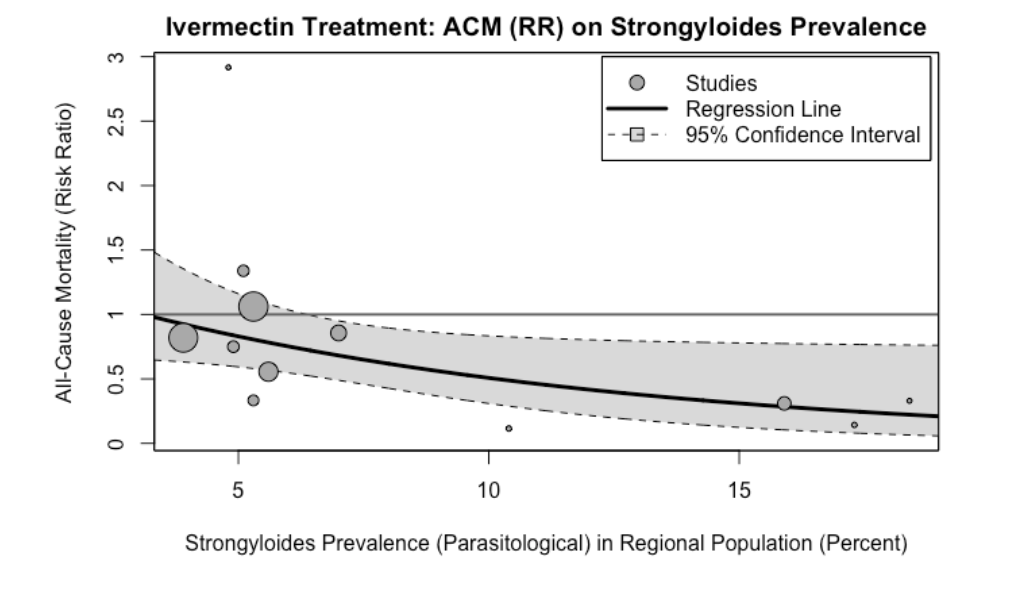
Excerpt:
About ten years ago, when the replication crisis started, we learned a certain set of tools for examining studies.Check for selection bias. Distrust “adjusting for confounders”. Check for p-hacking and forking paths. Make teams preregister their analyses. Do forest plots to find publication bias. Stop accepting p-values of 0.049. Wait for replications. Trust reviews and meta-analyses, instead of individual small studies.
These were good tools. Having them was infinitely better than not having them. But even in 2014, I was writing about how many bad studies seemed to slip through the cracks even when we pushed this toolbox to its limits. We needed new tools.
I think the methods that Meyerowitz-Katz, Sheldrake, Heathers, Brown, Lawrence and others brought to the limelight this year are some of the new tools we were waiting for.
Part of this new toolset is to check for fraud. About 10 – 15% of the seemingly-good studies on ivermectin ended up extremely suspicious for fraud. Elgazzar, Carvallo, Niaee, Cadegiani, Samaha. There are ways to check for this even when you don’t have the raw data. Like:
The Carlisle-Stouffer-Fisher method: Check some large group of comparisons, usually the Table 1 of an RCT where they compare the demographic characteristics of the control and experimental groups, for reasonable p-values. Real data will have p-values all over the map; one in every ten comparisons will have a p-value of 0.1 or less. Fakers seem bad at this and usually give everything a nice safe p-value like 0.8 or 0.9.
GRIM – make sure means are possible given the number of numbers involved. For example, if a paper reports analyzing 10 patients and finding that 27% of them recovered, something has gone wrong. One possible thing that could have gone wrong is that the data are made up. Another possible thing is that they’re not giving the full story about how many patients dropped out when. But something is wrong.
But having the raw data is much better, and lets you notice if, for example, there are just ten patients who have been copy-pasted over and over again to make a hundred patients. Or if the distribution of values in a certain variable is unrealistic, like the Ariely study where cars drove a number of miles that was perfectly evenly distributed from 0 to 50,000 and then never above 50,000.
Author(s): Scott Alexander
Publication Date: 17 Nov 2021
Publication Site: Astral Codex Ten at substack